Data farming is a process of collecting and analyzing data from various sources to gain valuable insights and inform decision-making. It involves using data mining, machine learning, and other data analysis techniques to uncover patterns, trends, and other valuable information that can be used to improve business processes, identify new opportunities, and mitigate risks.
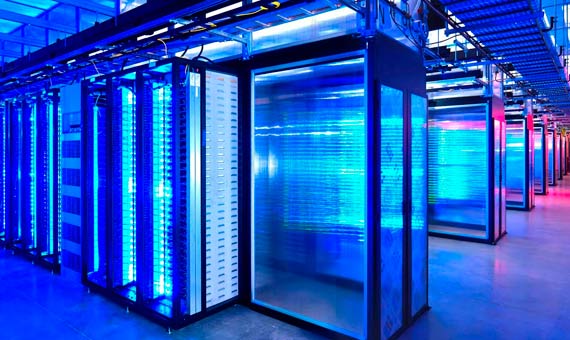
Data farming is used in a wide range of industries, including finance, healthcare, retail, and manufacturing. For example, in finance, data farming can be used to analyze market trends and make investment decisions. In healthcare, it can be used to analyze patient data and identify patterns that can be used to develop new treatments and improve patient outcomes. In retail, data farming can be used to analyze customer behavior and preferences, which can inform product development and marketing strategies.
One of the key benefits of data farming is that it enables organizations to make data-driven decisions. By collecting and analyzing data, organizations can gain a deeper understanding of their customers, products, and markets, which can inform strategic decision-making. For example, data farming can be used to identify which products are most popular with customers, which marketing channels are most effective, and which areas of the business are most profitable.
Data farming typically involves several steps. The first step is to identify the data sources that are relevant to the problem or opportunity being addressed. This may include internal data sources, such as sales and customer data, as well as external data sources, such as market data and social media data. Once the data sources have been identified, the data is collected and stored in a database or data warehouse.
The next step is to preprocess the data, which involves cleaning and preparing the data for analysis. This may involve removing duplicates, filling in missing data, and transforming the data into a format that can be used for analysis. Once the data has been preprocessed, it can be analyzed using various techniques, such as data mining and machine learning.
Data mining involves identifying patterns and trends in the data, which can be used to inform decision-making. For example, data mining can be used to identify which products are most popular with customers, which marketing channels are most effective, and which areas of the business are most profitable.
Machine learning is a type of artificial intelligence that involves training algorithms to make predictions based on historical data. For example, machine learning can be used to predict which customers are most likely to churn, which products are most likely to sell, and which marketing campaigns are most likely to be successful.
One of the key challenges of data farming is ensuring that the data is accurate and reliable. This requires careful data management and quality control, as well as appropriate data governance policies and procedures. It is also important to ensure that the data is properly secured to protect against data breaches and other security threats.
In conclusion, data farming is a powerful tool for organizations that want to make data-driven decisions. By collecting and analyzing data from various sources, organizations can gain a deeper understanding of their customers, products, and markets, which can inform strategic decision-making. However, it is important to ensure that the data is accurate, reliable, and properly secured to protect against data breaches and other security threats.